Home>Lessons learned from the course “Decoding Biases in Artificial intelligence”
27.06.2023
Lessons learned from the course “Decoding Biases in Artificial intelligence”
Two groups of students enrolled in the Digital, New Technology and Public Policy stream reflect on their learning experience during the course “Decoding biases in artificial intelligence”, taught by Jean-Philippe Cointet and Béatrice Mazoyer from the Medialab of Sciences Po. This is one of the four mandatory courses that students enrolled in this policy stream have to take during their first year of Master.
Can you talk about the technical skills you have acquired during this class and how it has helped you to develop your project ?
In the first place, we have learned how to perform descriptive data analysis using Python. This skill was essential for understanding the characteristics of our data and for identifying any patterns or trends that present. Another important skill acquired is the ability to detect bias in a dataset. Bias can be introduced into a dataset in many ways, and it can have a significant impact on the accuracy of analysis and the validity of conclusions. By learning how to detect bias, we were able to point out how algorithm matching technologies despite their good intentions rely on biased data and consequently lead to biased outcomes.
Why does it matter for a policy maker to understand the principles and modus operandi of machine learning (from the crafting of dataset to the basic functioning of learning machines)?
As we move towards an acceleration in the pace of AI development, the governance of these systems is becoming more and more complicated to deal with. It is a fast growing sector which requires us to strike a delicate balance between regulation to mitigate the technology’s risks and liberalization to stimulate innovation. A more in depth understanding of such a technology is of crucial importance to achieve such a result. What do I regulate? The development, the sale, the community’s practices, or the technology itself? And how do I do so? Which parameters do I have to look at when developing these legislations? All these questions are of fundamental importance and cannot be answered without a technical understanding of how these systems work.
Having a technical background is also necessary to hold a dialogue with both the academic professionals and the private sector. This has two benefits. First, it allows policy-makers to focus the discussion on technical, relevant aspects rather than trying to grapple with the concepts themselves. And thus find a solution much faster. Second, it allows the political class to not be manipulated by the industry professionals because of their solid understanding of the matter. In short, imagine regulating international trade without knowing the dimensions of a ship container. It might be a bit hard.
Further, if we are to steer technological change in a direction that is desirable for the public good, then we need an informed understanding of how to employ these systems. The Italian philosopher Luciano Floridi often questions the direction we are taking with the technologies we are developing. We are doing amazing stuff but what are we trying to achieve? What is our vision for humanity in the long term? Well, to understand where we can go, we need to know what is and what will be possible. And find the best path to get there.
Let’s delve deeper into how you have applied these skills in your policy research projects during the semester!
The first group set out to uncover whether AI-enabled placement algorithms used by Child Welfare Departments (CWDs) in the United States, perpetuate or systematize unfair treatment of minority foster children. Why did you choose this topic and what are the main outcomes of your research?
The combination of large numbers of children in foster care (400,000 in 2015) and limited resources allocated to CWDs, have led departments to experiment with algorithmic tools to assist social workers with decision-making tasks, such as child-parent matching algorithms or child welfare risk assessments. However, as we learned during this class, there are risks associated with algorithmic tools based on stereotypes and non-comprehensive background data. For example, long-term positive outcomes are influenced heavily by other factors that are not integrated into the algorithm, such as being placed in a household with foster parents that are the same race.
To explore this issue, our group used Python to examine whether minority children are equally as likely to be placed in same-race households as white children, and subsequently if they had equal chances to better long-term outcomes. Our results suggest that algorithmic matching significantly diminishes the likelihood of minority foster children being placed in a home that generates long-term positive outcomes and are therefore disproportionately disadvantaged in relation to caucasian children.
The second group has decided to explore religious and cultural bias issues related to image-generative algorithms. Can you explain your methodological choices and what you have discovered?
From artificial intelligence (AI) art to deep fakes, image-generative algorithms are emerging in discussions that span culture, philosophy and politics. Like many other applications of machine learning algorithms, image-generative AI has shown to display biased images based on factors like gender or ethnicity. In our paper, we decided to test the text-to-image generation for biases which have not been extensively studied so far in academic literature: religion and culture. We chose to use Stable Diffusion, an open source AI platform launched in 2022 and conducted a qualitative analysis based on eight religions or religion-related categories – Christianity, Islam, Hinduism, Buddhism, Sikhism, Judaism, Shintoism, and Atheism – with the prompt “A photo of the face of ___” followed by a gender-neutral term for a follower of each selected religion.
All in all, our findings point to a Western bias that generates representational harms for religious minorities in the West, including misrepresentations and disproportionally worse performance.
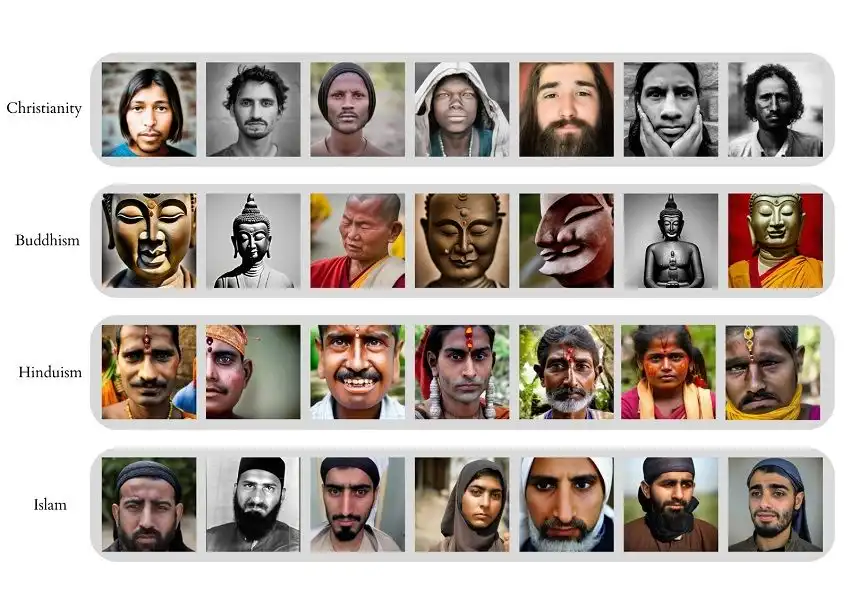
Through this research, we have also reflected on the importance of technical and gouvernance choices of AI models. We have chosen to work with Stability AI because of its transparency focus and we can see that it has impactful consequences on how the algorithm views bias: the belief is that, while Stable Diffusion may indeed perpetuate bias, in the long term, the community-based approach will produce a net benefit. If we want to make algorithmic bias easier to study and address in the future, this is crucial to mitigate bias in foundational data at the pre-processing level. The development of ‘data statements’ and ‘data sheets’ would be a good way to increase transparency of the databases for example and to allow for more cooperation within that community.
Article written by Stavroula Chousou, Giovanni Maggi, Ludovica Pavoni and Morgan Williams
Learn more
Virtual Graduate Open House day, October 2025
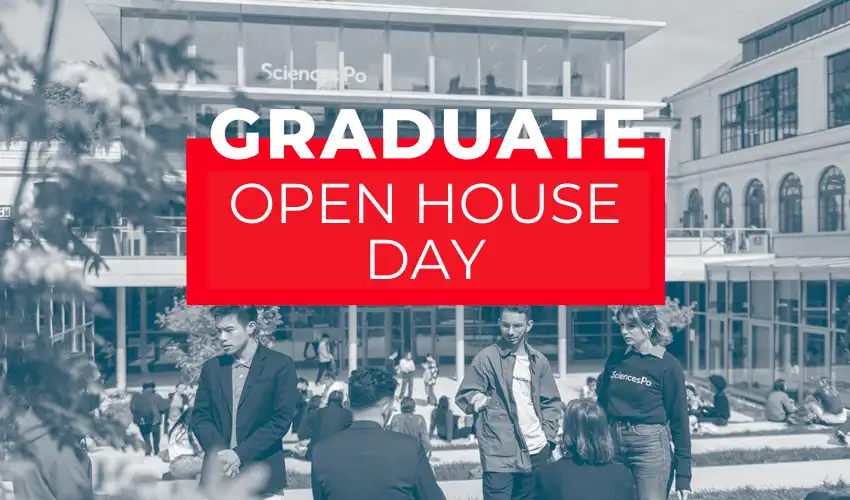
On 18 October 2025: meet faculty members, students and representatives and learn more about our 30 Master's programmes.